TLDR: There are too many technical vulnerabilities and there is too little organizational context for IT teams to patch effectively. Attack path modelling provides the organizational context, allowing security teams to prioritize vulnerabilities. The result is a system where CVEs can be parsed in, organizational context added, and attack paths considered, ultimately providing a prioritized list of vulnerabilities that need to be patched.
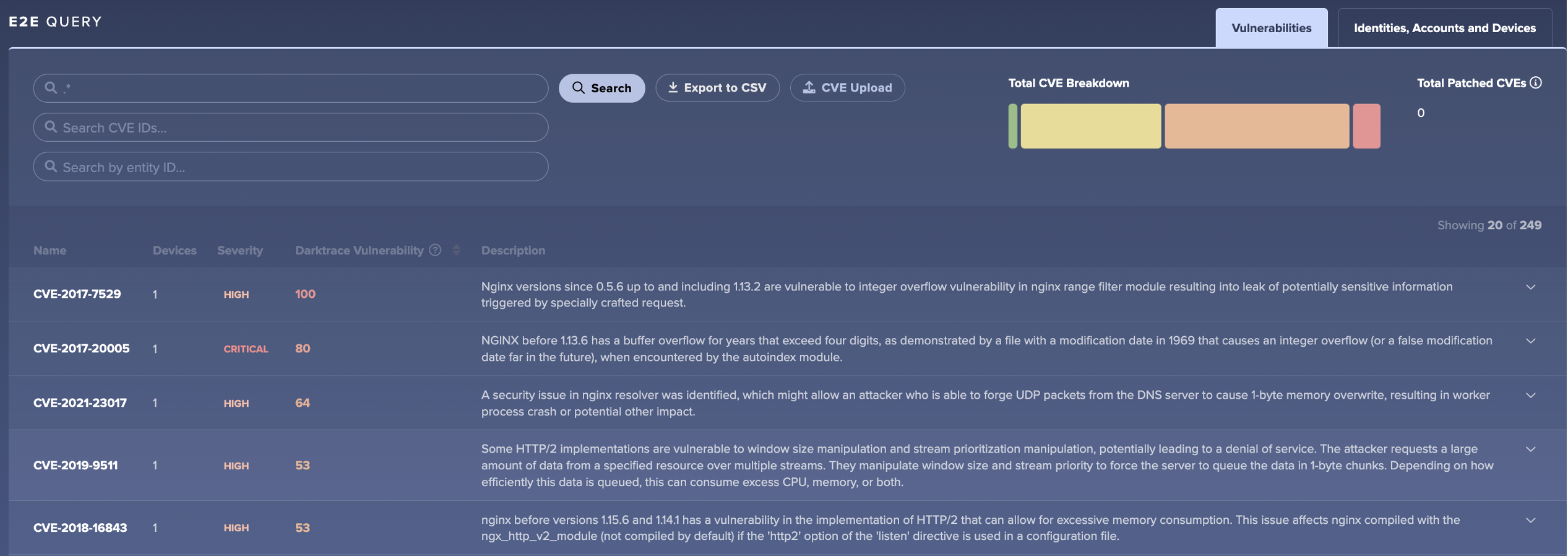
This blog post explains how Darktrace addresses the challenge of vulnerability prioritization. Most of the industry focusses on understanding the technical impact of vulnerabilities globally (‘How could this CVE generally be exploited? Is it difficult to exploit? Are there pre-requisites to exploitation? …’), without taking local context of a vulnerability into account. We’ll discuss here how we create that local context through attack path modelling and map it to technical vulnerability information. The result is a stunningly powerful way to prioritize vulnerabilities.
We will explore:
1) The challenge and traditional approach to vulnerability prioritization
2) Creating local context through machine learning and attack path modelling
3) Examining the result – contextualized, vulnerability prioritization
The Challenge
Anyone dealing with Threat and Vulnerability Management (TVM) knows this situation:
You have a vulnerability scanning report with dozens or hundreds of pages. There is a long list of ‘critical’ vulnerabilities. How do you start prioritizing these vulnerabilities, assuming your goal is reducing the most risk?
Sometimes the challenge is even more specific – you might have 100 servers with the same critical vulnerability present (e.g. MoveIT). But which one should you patch first, as all of those have the same technical vulnerability priority (‘critical’)? Which one will achieve the biggest risk reduction (critical asset e.g.)? Which one will be almost meaningless to patch (asset with no business impact e.g.) and thus just a time-sink for the patch and IT team?
There have been recent improvements upon flat CVE-scoring for vulnerability prioritization by adding threat-intelligence about exploitability of vulnerabilities into the mix. This is great, examples of that additional information are Exploit Prediction Scoring System (EPSS) and Known Exploited Vulnerabilities Catalogue (KEV).

With CVE and CVSS scores we have the theoretical technical impact of vulnerabilities, and with EPSS and KEV we have information about the likelihood of exploitation of vulnerabilities. That’s a step forward, but still doesn’t give us any local context. Now we know even more about the global and generic technical risk of a vulnerability, but we still lack the local impact on the organization.
Let’s add that missing link via machine learning and attack path modelling.
Adding Attack Path Modelling for Local Context
To prioritize technical vulnerabilities, we need to know as much as we can about the asset on which the vulnerability is present in the context of the local organization. Is it a crown jewel? Is it a choke point? Does it sit on a critical attack path? Is it a dead end, never used and has no business relevance? Does it have organizational priority? Is the asset used by VIP users, as part of a core business or IT process? Does it share identities with elevated credentials? Is the human user on the device susceptible to social engineering?
Those are just a few typical questions when trying to establish local context of an asset. Knowing more about the threat landscape, exploitability, or technical information of a CVE won’t help answer any of the above questions. Gathering, evaluating, maintaining, and using this local context for vulnerability prioritization is the hard part. This local context often resides informally in the head of the TVM or IT team member, having been assembled by having been at the organization for a long time, ‘knowing’ systems, applications and identities in question and talking to asset and application owners if time permits. This does unfortunately not scale, is time-consuming and heavily dependent on individuals.
Understanding all attack paths for an organization provides this local context programmatically.
We discover those attack paths, and these are bespoke for each organization through Darktrace PREVENT™, using the following method (simplified):
1) Build an adaptive model of the local business. Collect, combine, and analyze (using machine learning and non-machine learning techniques) data from various data domains:
a. Network, Cloud, IT, and OT data (network-based attack paths, communication patterns, peer-groups, choke-points, …). Natively collected by Darktrace technology.
b. Email data (social engineering attack paths, phishing susceptibility, external exposure, security awareness level, …). Natively collected by Darktrace technology.
c. Identity data (account privileges, account groups, access levels, shared permissions, …). Collected via various integrations, e.g. Active Directory.
d. Attack surface data (internet-facing exposure, high-impact vulnerabilities, …). Natively collected by Darktrace technology.
e. SaaS information (further identity context). Natively collected by Darktrace
f. Vulnerability information (CVEs, CVSS, EPSS, KEV, …). Collected via integrations, e.g. Vulnerability Scanners or Endpoint products.
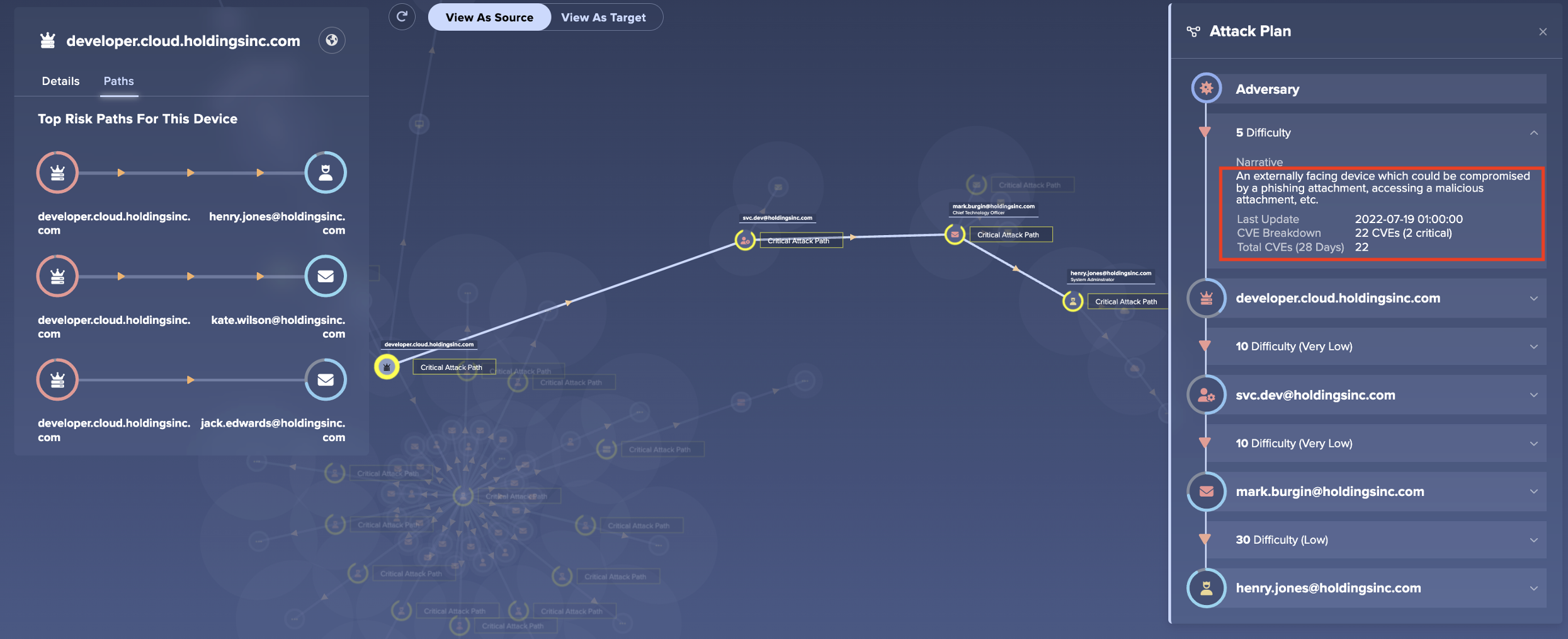
2) Understand what ‘crown jewels’ are and how to get to them. Calculate entity importance (user, technical asset), exposure levels, potential damage levels (blast radius) weakness levels, and other scores to identify most important entities and their relationships to each other (‘crown jewels’).
Various forms of machine learning and non-machine learning techniques are used to achieve this. Further details on some of the exact methods can be found here. The result is a holistic, adaptive and dynamic model of the organization that shows most important entities and how to get to them across various data domains.
The combination of local context and technical context, around the severity and likelihood of exploitation, creates the Darktrace Vulnerability Score. This enables effective risk-based prioritisation of CVE patching.
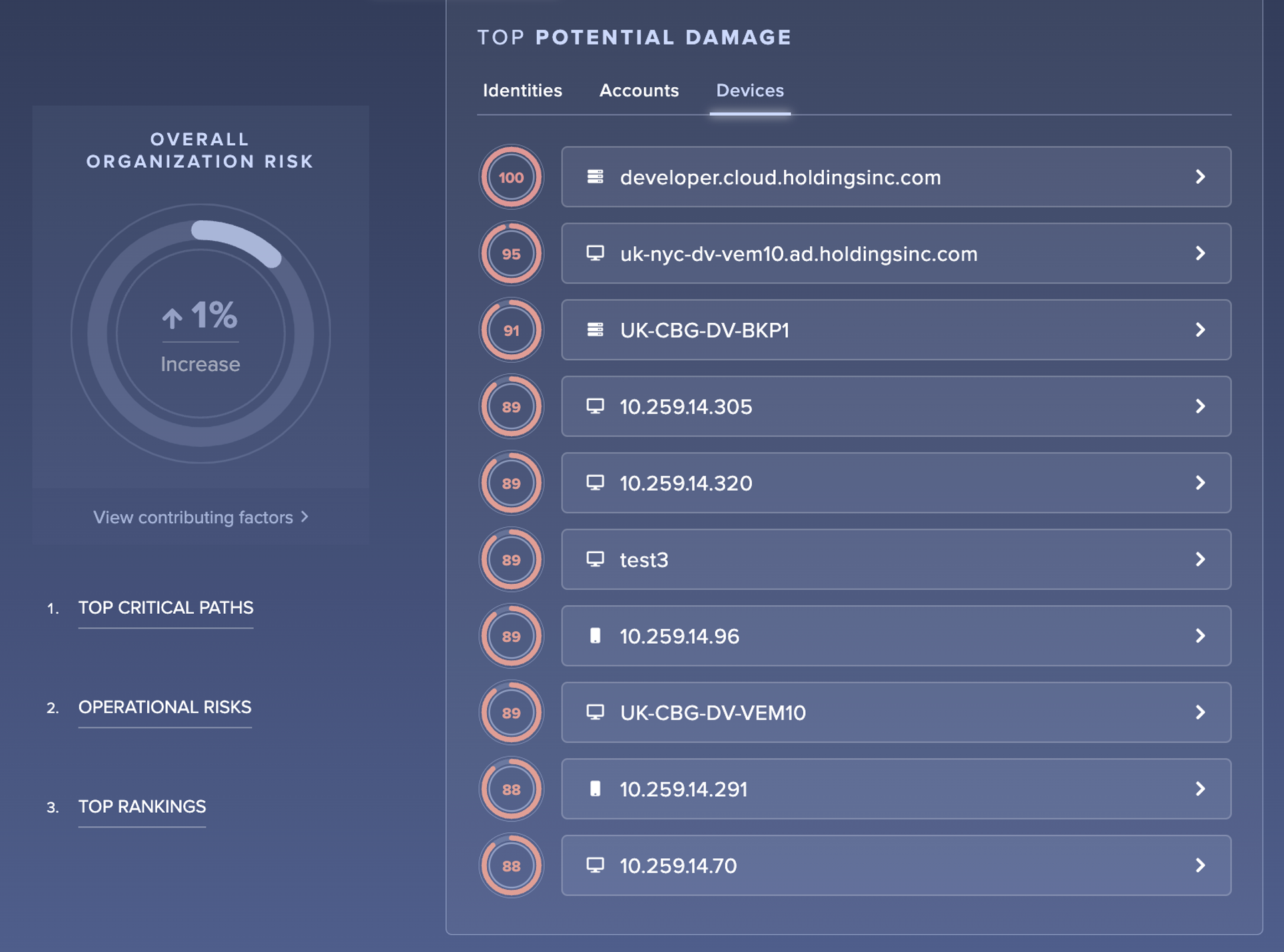
3) Map the attack path model of the organization to common cyber domain knowledge. We can then combine things like MITRE ATT&CK techniques with those identified connectivity patterns and attack paths – making it easy to understand which techniques, tools and procedures (TTPs) can be used to move through the organization, and how difficult it is to exploit each TTP.
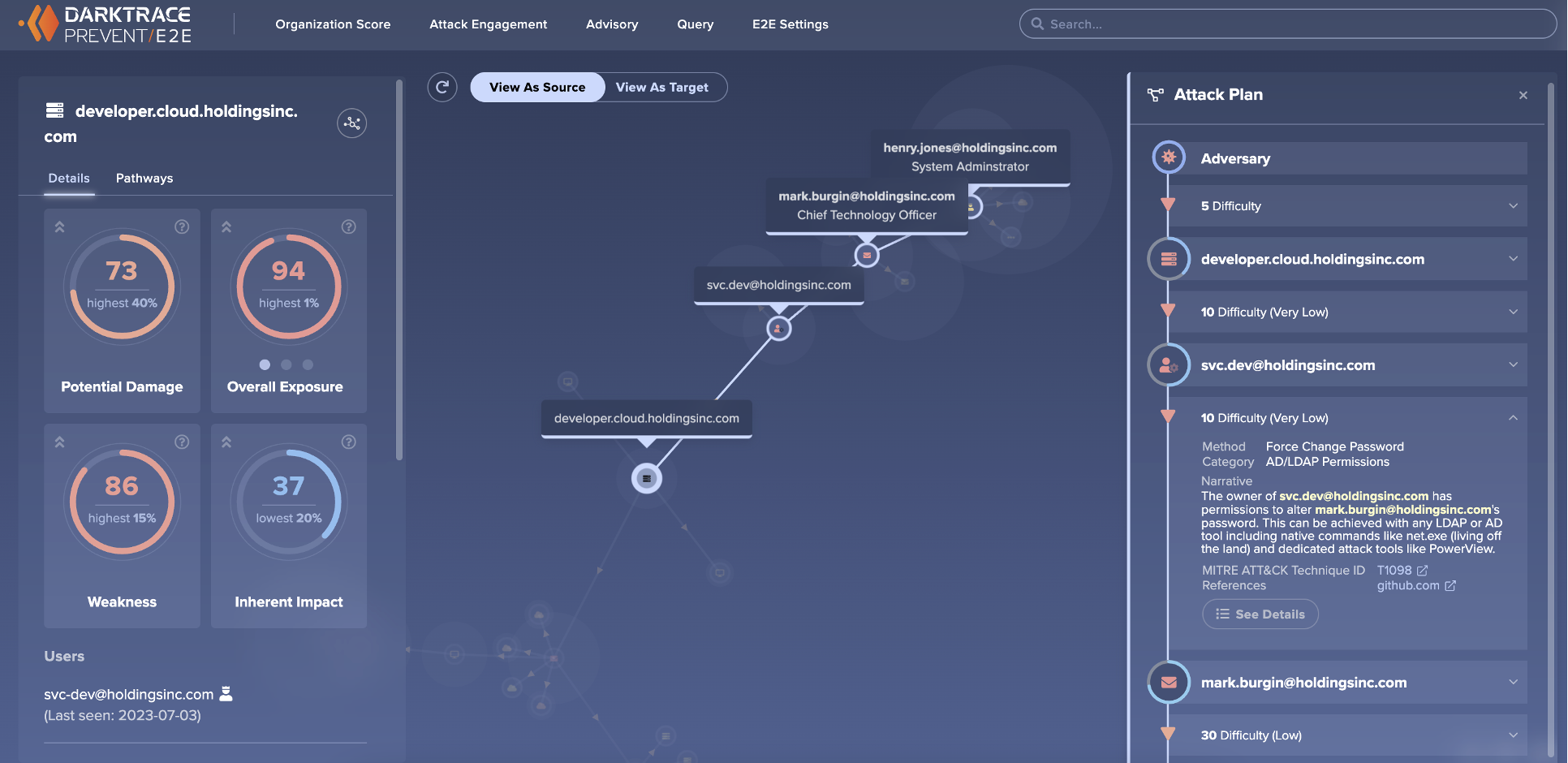
We can now easily start prioritizing CVE patching based on actual, organizational risk and local context.
Bringing It All Together
Finally, we overlay the attack paths calculated by Darktrace with the CVEs collected from a vulnerability scanner or EDR. This can either happen as a native integration in Darktrace PREVENT, if we are already ingesting CVE data from another solution, or via CSV upload.
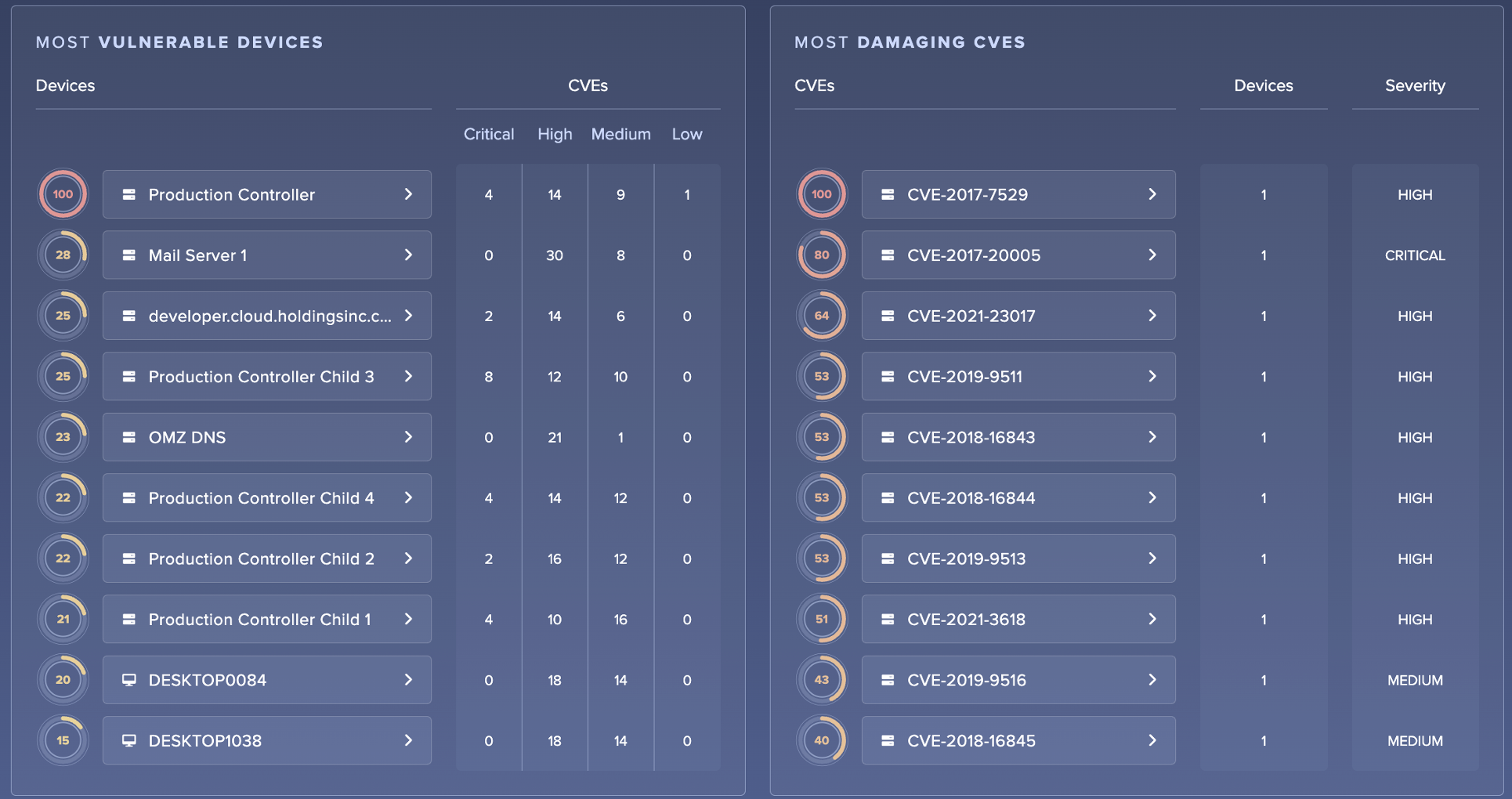
But you can also go further than just looking at the CVE that delivers the biggest risk reduction globally in your organization if it is patched. You can also look only at certain group of vulnerabilities, or a sub-set of devices to understand where to patch first in this reduced scope:
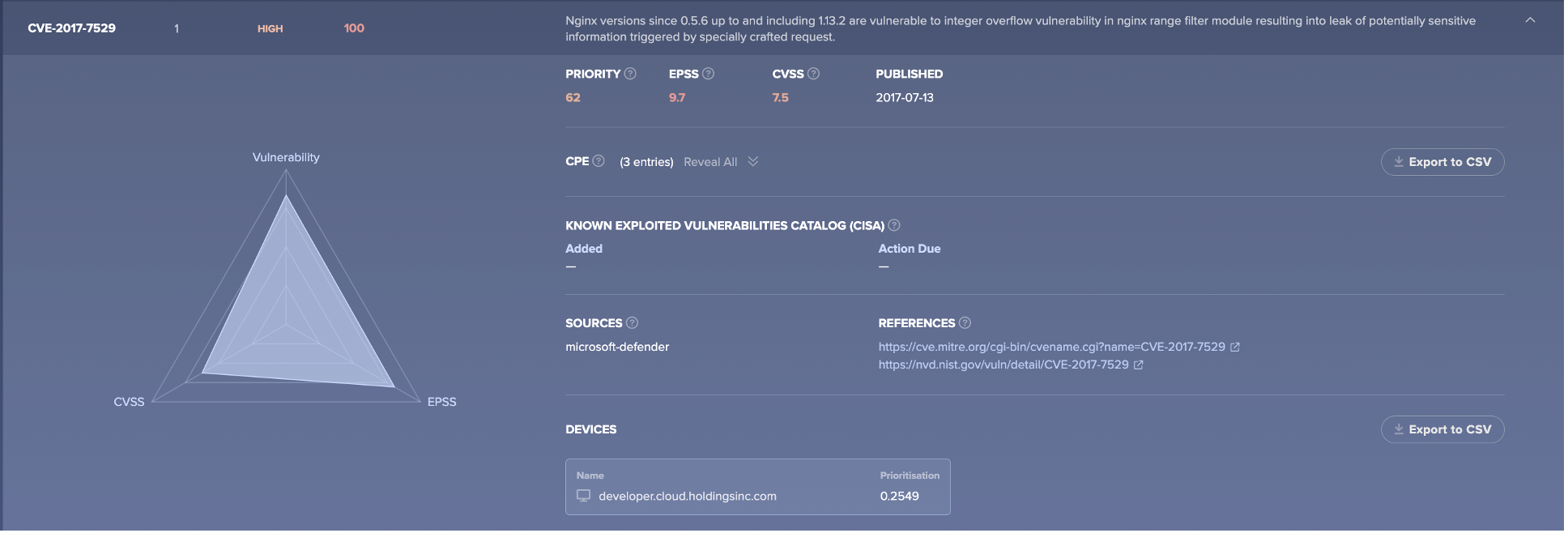
This also provides the TVM team clear justification for the patch and infrastructure teams on why these vulnerabilities should be prioritized and what the positive impact will be on risk reduction.
Attack path modelling can be utilized for various other use cases, such as threat modelling and improving SOC efficiency. We’ll explore those in more depth at a later stage.
Want to explore more on using machine learning for vulnerability prioritization? Want to test it on your own data, for free? Arrange a demo today.