In diesem Blog werfen wir einen Blick auf die Log4Shell-Schwachstelle und stellen Beispiele aus der Praxis vor, wie Darktrace Angriffe, die versuchen, Log4Shell in freier Wildbahn auszunutzen, erkennt und darauf reagiert.
Log4Shell ist jetzt der bekannte Name für CVE-2021-44228 - eine Zero-Day-Schwachstelle des Schweregrads 10, die ein bekanntes Java-Protokollierungsprogramm namens Log4j ausnutzt. Schwachstellen werden täglich entdeckt, und einige sind schwerwiegender als andere, aber die Tatsache, dass dieses Open-Source-Dienstprogramm in fast alles eingebettet ist, einschließlich der Mars Ingenuity-Drohne, macht diese Schwachstelle umso bedrohlicher. Details und weitere Updates zu Log4Shell sind zum Zeitpunkt der Veröffentlichung dieses Blogs noch in Arbeit.
Normalerweise werden Zero-Days, die in der Lage sind, so viele Systeme zu erreichen, geheim gehalten und nur von Nationalstaaten für hochrangige Ziele oder Operationen eingesetzt. Dieser Zero-Day wurde jedoch erst entdeckt, als er auf Minecraft-Spieleserver eingesetzt wurde und im Chat zwischen Spielern ausgetauscht wurde.
Es sollten zwar alle Schritte unternommen werden, um Abhilfemaßnahmen für die Log4Shell-Schwachstelle zu implementieren, aber dies kann einige Zeit dauern. Wie hier gezeigt, kann die Verhaltenserkennung verwendet werden, um nach Anzeichen für Aktivitäten nach der Ausnutzung der Schwachstelle zu suchen, z. B. Scannen, coin mining, lateral Movement und andere Aktivitäten.
Darktrace entdeckte zunächst die Log4Shell-Schwachstelle, die auf die Internet-Server eines unserer Kunden abzielt, wie Sie unten in einer aktuellen anonymisierten Bedrohungsuntersuchung im Detail sehen können. Diese wurde mithilfe des Cyber-AI-Analyst aufgedeckt, gemeldet und anschließend von unserem SOC-Team analysiert. Wichtig zu erwähnen ist, dass hierbei bereits vorhandene Algorithmen verwendet wurden, ohne dass die Klassifizierer neu trainiert oder die Reaktionsmechanismen als Reaktion auf die Log4Shell-Cyberangriffe angepasst wurden.
Wie Log4Shell funktioniert
Die Schwachstelle funktioniert, indem sie eine unsachgemäße Eingabevalidierung durch das Java Naming and Directory Interface (JNDI) ausnutzt. Ein Befehl kommt von einem HTTP-User-Agent, einer verschlüsselten HTTPS-Verbindung oder sogar einer Chatroom-Nachricht. Die JNDI sendet ihn an das Zielsystem, wo er ausgeführt wird. Die meisten Libraries und Applikationen verfügen über Prüfungen und Schutzmechanismen, um dies zu verhindern, aber wie hier zu sehen ist, werden diese manchmal übersehen.
Verschiedene Bedrohungsakteure haben damit begonnen, die Schwachstelle für Angriffe zu nutzen, die von wahllosen Krypto-Mining-Kampagnen bis hin zu gezielten, komplexen Angriffen reichen.
Reales Beispiel 1: Log4Shell wird am CVE-ID-Veröffentlichungsdatum ausgenutzt
Darktrace sah dieses erste Beispiel am 10. Dezember, dem gleichen Tag, an dem die CVE-ID veröffentlicht wurde. Wir sehen oft, dass öffentlich dokumentierte Schwachstellen innerhalb weniger Tage von Bedrohungsakteuren als Waffe eingesetzt werden. Dieser Angriff betraf ein dem Internet zugewandten Gerät in der demilitarisierten Zone (DMZ) eines Unternehmens. Darktrace hatte den Server aufgrund seines Verhaltens automatisch als dem Internet zugewandtes Gerät eingestuft.
Das Unternehmen hatte Darktrace im On-Prem-Netz als einen von vielen Abdeckungsbereichen implementiert, zu denen auch Cloud, E-Mail und SaaS gehören. Bei diesem Einsatz hatte Darktrace einen guten Überblick über den DMZ-Verkehr. Antigena war in dieser Umgebung nicht aktiv, Darktrace befand sich nur im Erkennungsmodus. Trotz dieser Tatsache war der betreffende Kunde in der Lage, diesen Vorfall innerhalb weniger Stunden nach dem ersten Alarm zu erkennen und zu beheben. Der Angriff war automatisiert und hatte zum Ziel, einen als Kinsing bekannten Krypto-Miner einzusetzen.
Bei diesem Angriff erschwerte der Angreifer die Entdeckung der Kompromittierung, indem er die anfängliche Befehlsinjektion verschlüsselte und HTTPS anstelle des in der Praxis üblichen HTTP verwendete. Obwohl mit dieser Methode herkömmliche Regeln und signaturbasierte Systeme umgangen werden konnten, war Darktrace in der Lage, mehrere ungewöhnliche Verhaltensweisen Sekunden nach der ersten Verbindung zu erkennen.
Erste Details der Kompromitierung
Durch Peer-Analysen hatte Darktrace zuvor erfahren, was dieses spezielle DMZ-Gerät und seine Peer-Gruppe normalerweise in der Umgebung tun. Während des ersten Angriffs entdeckte Darktrace verschiedene subtile Anomalien, die zusammengenommen den Angriff offensichtlich machten.
- 15:45:32 Eingehende HTTPS-Verbindung zum DMZ-Server von seltener russischer IP - 45.155.205[.]233;
- 15:45:38 Der DMZ-Server stellt eine neue ausgehende Verbindung zu derselben seltenen russischen IP-Adresse her und verwendet dabei zwei neue Benutzeragenten: Java-Benutzer-Agent und Curl über einen Port, der im Vergleich zum bisherigen Verhalten ungewöhnlich ist, um HTTP zu bedienen;
- 15:45:39 Der DMZ-Server verwendet eine HTTP-Verbindung mit einem anderen neuen curl-Benutzeragenten ('curl/7.47.0') zur gleichen russischen IP. Der URI enthält Aufklärungsinformationen vom DMZ-Server.
All diese Aktivitäten wurden nicht entdeckt, weil Darktrace sie schon einmal gesehen hatte, sondern weil sie stark vom normalen "Verhaltensmuster" für diesen und ähnliche Server in dieser speziellen Organisation abwichen.
Dieser Server hat sich mit seltenen IP-Adressen im Internet verbunden, er hat über Protokoll- und Port-Kombinationen, die er nie benutzt, nie zuvor benutzte User-Agents verwendet. Jede einzelne Anomalie mag für sich genommen ein leicht ungewöhnliches Verhalten gezeigt haben - aber zusammengenommen und im Kontext dieses speziellen Geräts und dieser Umgebung analysiert, erzählen die Entdeckungen eindeutig eine größere Geschichte eines laufenden Cyberangriffs.
Darktrace hat diese Aktivität mit verschiedenen Modellen entdeckt:
- Anomalous Connection / New User Agent to IP Without Hostname
- Anomalous Connection / Callback on Web Facing Device
Weitere Werkzeuge und Crypto-Miner Download
Weniger als 90 Minuten nach der ersten Kompromittierung begann der infizierte Server, bösartige Skripte und ausführbare Dateien von einer seltenen ukrainischen IP 80.71.158[.]12 herunterzuladen.
Die folgenden Nutzerdaten wurden anschließend der Reihe nach von der ukrainischen IP heruntergeladen:
- hXXp://80.71.158[.]12//lh.sh
- hXXp://80.71.158[.]12/Expl[REDACTED].class
- hXXp://80.71.158[.]12/kinsing
- hXXp://80.71.158[.]12//libsystem.so
- hXXp://80.71.158[.]12/Expl[REDACTED].class
Ohne Bedrohungsdaten oder Erkennungen, die auf statischen Kompromittierungsindikatoren (IoC) wie IPs, Domänennamen oder Datei-Hashes basieren, erkannte Darktrace diesen nächsten Schritt des Angriffs in Echtzeit.
Der betreffende DMZ-Server hat in der Vergangenheit nie mit dieser ukrainischen IP-Adresse über diese ungewöhnlichen Ports kommuniziert. Es ist auch höchst ungewöhnlich, dass dieses Gerät und seine Gegenspieler Skripte oder ausführbare Dateien dieser Art von einer externen Destination auf diese Weise herunterladen. Kurz nach diesen Downloads begann der DMZ-Server, Krypto-Mining zu betreiben.
Darktrace hat diese Aktivität mit verschiedenen Modellen entdeckt:
- Anomalous File / Script from Rare External Location
- Anomalous File / Internet Facing System File Download
- Device / Internet Facing System with High Priority Alert
Sofortige Aufdeckung des Log4Shell-Vorfalls
Neben Darktrace, das jeden einzelnen Schritt dieses Angriffs in Echtzeit erkennt, hat der Cyber-AI-Analyst auch den übergreifenden Sicherheitsvorfall, der eine zusammenhängende Erzählung für den Gesamtangriff enthält, als den Vorfall mit der höchsten Priorität, innerhalb einer Woche von Vorfällen und Warnungen, auf Darktrace aufgedeckt. Das bedeutet, dass dieser Vorfall das offensichtlichste und unmittelbarste Element war, auf das die Sicherheitsteams aufmerksam wurden, als er sich entwickelte. Der Cyber-AI-Analyst fand jede Phase dieses Vorfalls und stellte genau die Fragen, die Sie von Ihren menschlichen SOC-Analysten erwarten würden. Der vom Cyber-AI-Analysten erstellte Bericht in natürlicher Sprache enthält eine Zusammenfassung der einzelnen Phasen des Vorfalls, gefolgt von den wichtigen Daten, die menschliche Analysten benötigen, in einem leicht verständlichen Format. Jede Registerkarte steht für einen anderen Teil des Vorfalls und beschreibt die tatsächlichen Schritte, die während des jeweiligen Untersuchungsprozesses unternommen wurden.
Das Ergebnis ist, dass man sich nicht mehr durch Low-Level-Warnungen wühlen muss, dass man keine punktuellen Erkennungen mehr triagieren muss, dass man die Erkennungen nicht mehr in einen größeren Vorfallskontext einordnen muss und dass man keinen Bericht mehr schreiben muss. All dies wurde automatisch vom KI-Analysten erledigt und spart den menschlichen Teams wertvolle Zeit.
Der nachstehende Vorfallsbericht wurde automatisch erstellt und konnte als PDF-Datei in verschiedenen Sprachen heruntergeladen werden.
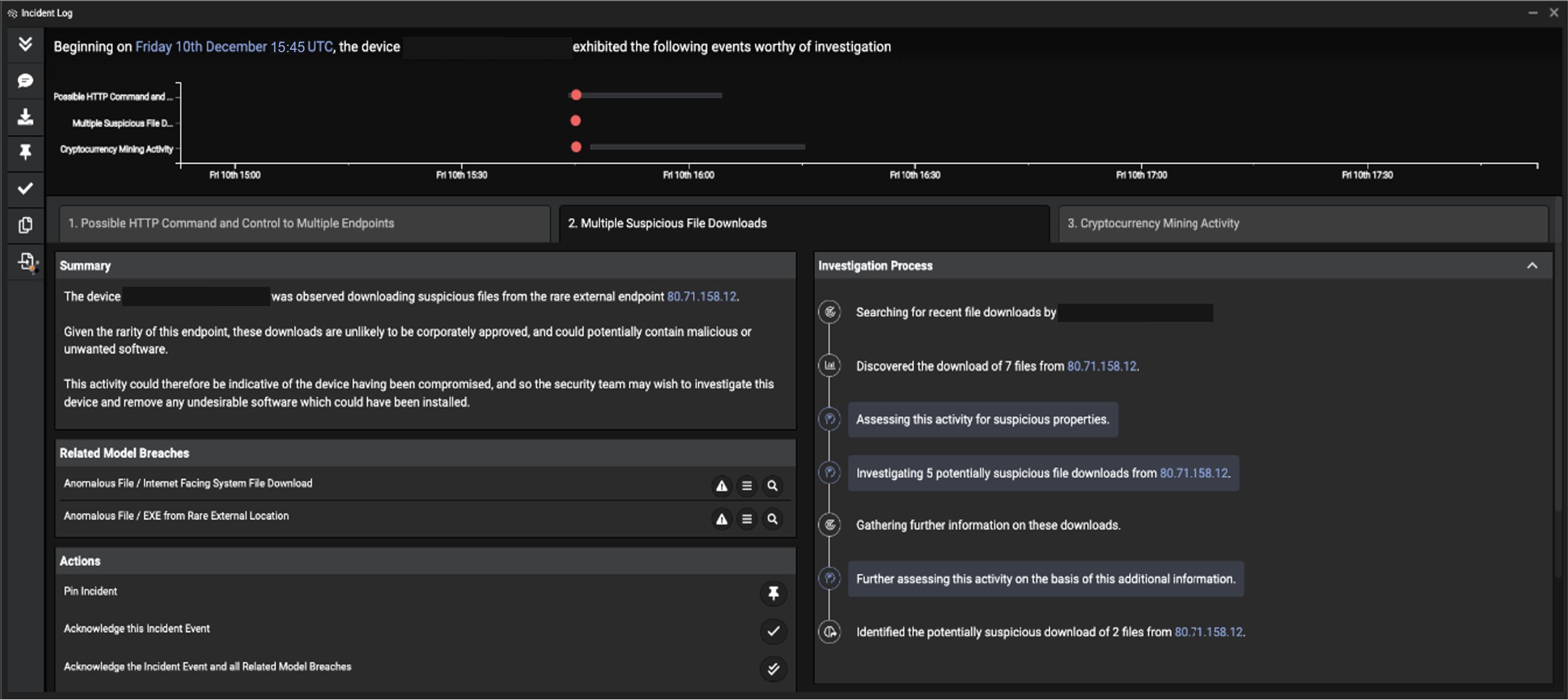
Abbildung 1: Der Cyber AI Analyst von Darktrace zeigt mehrere Stufen des Angriffs und erklärt den Untersuchungsprozess
Beispiel aus der Praxis 2: Reaktion auf einen anderen Angriff durch Log4Shell
Am 12. Dezember wurde der Internet-Server eines anderen Unternehmens zunächst über Log4Shell kompromittiert. Die Details der Kompromittierung sind zwar anders - es sind andere IoCs beteiligt - aber Darktrace hat den Angriff ähnlich wie im ersten Beispiel erkannt und analysiert.
Interessanterweise hatte diese Organisation Darktrace Antigena im autonomen Modus auf ihrem Server, was bedeutet, dass die KI eigenständig Maßnahmen ergreifen kann, um auf laufende Cyberangriffe zu reagieren. Diese Reaktionen können über eine Vielzahl von Mechanismen erfolgen, z. B. über API-Interaktionen mit Firewalls, anderen Sicherheitstools oder native Reaktionen von Darktrace.
Bei diesem Angriff wurde die seltene externe IP 164.52.212[.]196 für die Command-and-Control (C2)-Kommunikation und die Verbreitung von Malware verwendet, wobei HTTP über Port 88 genutzt wurde, was für dieses Gerät, diese Peer Group und diese Organisation höchst ungewöhnlich war.
Antigena reagierte in dieser Organisation in Echtzeit, basierend auf dem spezifischen Kontext des Angriffs, ohne dass ein Mensch involviert war. Antigena interagierte in diesem Fall mit der Firewall des Unternehmens, um alle Verbindungen zu oder von der bösartigen IP-Adresse - in diesem Fall 164.52.212[.]196 - über Port 88 für zwei Stunden zu blockieren, mit der Option, die Blockierung und die Dauer zu verlängern, wenn der Angriff anscheinend andauert. Dies ist in der nachstehenden Abbildung zu sehen:

Abbildung 2: Die Reaktion der Antigena
Jetzt kommt der Clou: Dank selbstlernender KI weiß Darktrace genau, was der Server im Internet normalerweise tut und was nicht, bis hin zu jedem einzelnen Datenpunkt. Aufgrund der verschiedenen Anomalien ist sich Darktrace sicher, dass es sich um einen schweren Cyberangriff handelt.
Antigena greift nun ein und erzwingt den regulären Ablauf des Lebens dieses Servers in der DMZ. Das bedeutet, dass der Server weiterhin tun kann, was er normalerweise tut - aber alle höchst anomalen Aktionen werden unterbrochen, wenn sie in Echtzeit stattfinden, wie z. B. die Kommunikation mit einer seltenen externen IP über Port 88, die HTTP zum Herunterladen von ausführbaren Dateien dient.
Natürlich kann der Mensch die Sperre jederzeit ändern oder aufheben. Antigena kann auch so konfiguriert werden, dass es sich im Bestätigungsmodus befindet, d. h. der Mensch ist zu bestimmten Zeiten am Tag (z. B. während der Bürozeiten) oder zu jeder Zeit eingebunden, je nach den Bedürfnissen und Anforderungen einer Organisation.
Schlussfolgerung
Dieser Blog veranschaulicht weitere Aspekte von Cyberangriffen, die die Log4Shell-Schwachstelle ausnutzen. Außerdem wird gezeigt, wie Darktrace Zero-Day-Angriffe erkennt und auf sie reagiert, wenn Darktrace Einblick in die angegriffenen Entitäten hat.
Während Log4Shell die IT- und Sicherheitsnachrichten dominiert, sind ähnliche Schwachstellen in der Vergangenheit aufgetaucht und werden auch in Zukunft auftreten. Wir haben bereits über unseren Ansatz zur Erkennung und Reaktion auf ähnliche Schwachstellen und damit verbundene Cyberangriffe gesprochen:
- die jüngste Sicherheitslücke in Gitlab;
- die ProxyShell Exchange Server-Schwachstellen, als sie noch ein Zero-Day waren;
- und die Citrix Netscaler-Schwachstelle.
Wie immer sollten Unternehmen eine "Defense-in-Depth"-Strategie anstreben, die präventive Sicherheitskontrollen mit Erkennungs- und Reaktionsmechanismen sowie einem strengen Patch-Management kombiniert.
Vielen Dank an Brianna Leddy (Darktrace’s Director of Analysis) für ihre Erkenntnisse über die oben genannte Bedrohung.